Agentic AI Everywhere: The Future of Autonomous Intelligence?
Neetu Pathak: So having an autonomous
agent do something without check-in
balances, obviously it's not gonna work.
If you have the right check-in
balances, then it shouldn't
be that much of a problem.
The only difference though, is we
as humans, when we grow up, we have
a lot of environment conditioning.
Like the fact that we understand, well,
this is ethical and this is not ethical.
Drew Dimmick: And so when you get to doing
those kind of menial or travel planning,
like things, know, while it might've been
fun in the past when I had less stresses
on my life, it's not fun right now.
You just want to get it done and you
want something that truly understands,
you know, all of the things that you're
looking for and take care of that for you.
in our our resource constrained
environments, having something
like an assistant, being able to
do that for you reliably is super.
Attractive.
And I think that will be quite
beneficial for society in general
because there we don't have enough
people to get the work done.
Dan Mitchell: Welcome to mainly ai.
I'm Dan Mitchell and we're
joined by my co-host Viby Jacob.
Today we are going to
talk about ag agentic ai.
Alright so it's a really
timely topic here.
We're gonna talk about what are the
implications, where is it being applied?
it mean for the future?
First we'll get into the definition.
From there we'll talk
some real world use cases.
I personally wanna talk some tech about
some recent news on MCP and A two A, those
three letter acronyms and what those mean.
We'll get into ethical considerations.
You know, we talked a little bit
about I sent you a, a link to a paper
that suggests that maybe we shouldn't
have completely autonomous systems.
So have you weigh in on that.
And with that, I'd like
to welcome Neetu, Pathak.
She's CEO and co-founder of
SkyMel, and Drew Dimmick, CTO
and co-founder of Prompt 360.
Welcome.
Drew Dimmick: Thanks for having us.
Dan Mitchell: yeah.
So why don't we have you
introduce yourselves?
Ni why don't you go first?
Neetu Pathak: Yeah.
So I'm Neetu Pathak,
co-founder and CEO of SkyMel.
So what we're building is an orchestration
agent, what that means today.
So three or four years back, you
had a static pipeline of what model
to run because you trained it once
or tweakers once or twice a year.
What we are doing is we are deciding in
real time what the pipeline looks like
to run your AI and deliver it in runtime.
Dan Mitchell: Okay, great.
Great.
And Drew, tell us you know
a little bit about yourself.
You had an interesting job right before
you did this startup too, so you can
mention that and then what you're what
you're building, what you're doing.
Drew Dimmick: Sir, so Drew Dimick I
spent the last six and a half years.
As chief architect of a large financial
services company based in Toronto.
And there I got to see kind of the
beginning of what we started working on
here at Prompt 360, which is an agent
approach to help IT organizations do
research and respond to urgent matters
like audit or cyber vulnerabilities by
using a, an agent approach to go pull
data from lots of enterprise IT systems.
Dan Mitchell: Very
Very cool.
I love that, you know, there's so many
different use cases for it and I think
we're gonna have listeners from all
aspects of technology, some people
very technical and hands-on keyboard,
other people who are really just
trying to learn about AI in general.
And there's all this buzz
about agents and AG agentic.
So, I think where we start off
right is kind of the general
definition of ag agentic.
If I were to average out everything,
I searched on the internet, everything
the LLMs tell me about Ag agentic.
It's autonomous AI systems
that act independently.
In dynamic environments
to achieve specific goals.
Like that's a very academic definition
of what it is and what it does.
But you know, like, so we think
about agents, like what are some
of the things, Viby, that we
would think about in that case?
I.
Viby Jacob: I think the first one
is that the goals within an agent
system are set by the human right.
So we need to sort of be mindful of that.
agents themselves are goal directed,
meaning they strive to achieve the goal
set by the human without any specific
direction on how to achieve those, right?
So they go through a process of multi-step
reasoning, self-directed reasoning, and
they act autonomously, which is acting
independently to analyze the data, take
actions, draw conclusions, et cetera.
And then the third thing is
like they are context aware.
They interact with the environment
the tools, et cetera, and they're
capable of learning as well as
adapting from those interactions.
So those are what I would call as
like the three main characteristics.
Of an agent system, so they're just not
responding to a prompt, but actively
working towards the human directed
goal through a multi-step process.
Dan Mitchell: You guys
weigh in, Neetu, Drew,
Neetu Pathak: So
Dan Mitchell: right here?
Is this, is this accurate?
Neetu Pathak: I mean, it is, so
there are a lot of definition.
Of agents.
So what I did was just to kind
of figure out what would be
a 360 degree view of agents.
I started looking through
all the research paper.
So one of the things people don't realize
it, the term agent is not a new concept.
It started coming up in machine
learning research paper in nineties,
but the concept actually started
coming around sixties and seventeens.
Even if you look at so there,
there was a paper which was
talking about BID or it was PDI.
So it was belief, desire, and influence.
Yes, it was BDI.
So, um, that was like the first
concept of what we see today.
I recently wrote a guest article where
I kind of combined five different
actions that agent needs to do.
So first is called perceive.
That means you can see the
environment you are in.
And then reason I.
That means you can collect all the
data and kind of make sense of it.
What does it mean, what the intent is?
And also understand
anomalies and constraints.
One of the things people don't talk about
an agent is, oh, you have a goal, but you
still have to work within a constraint.
Like if I ask an agent, Hey, can you
book me a ticket from SF to India?
Obviously I'll have some
constraint in terms of money stops.
So people don't talk about that.
Every agent has to work within a
constraint, so that's important.
The next is obviously plan.
So now you have all the data, you
have kind of your constraints and
you go, uh, you have your goal.
Now you are coming up with different
decisions of how you can reach there.
And once you decide the right
path, then you have to act on it.
And once you act, then you ha need
to have an ability to monitor did you
do it right or did you do it wrong?
And if something went
wrong, what went wrong?
And you learn from it.
And it's a feedback loop.
So, and this is not my definition,
I just read a lot of paper to kind
of combine everything together.
What would a agent look like?
Dan Mitchell: Sure.
Sure.
Yeah.
Um, drew, you got anything to add to that?
Drew Dimmick: Yes.
Actually the great, uh, base definition
and I like your concept model.
Me too.
we believe the same thing,
or things in addition.
You know, we really are stressing the
memory and the embedded knowledge and
that we learn as we're going through
those processes because, that's where
the real value starts to come in.
For example, in our systems, we're
spending a lot of time learning
about topologies and it, subsystems.
And we are re remembering those so
that when the next user comes along
you're able to harvest that data.
for additional queries, and that
may be completely different intense,
but need the same source data.
So those the long-term memory and
then the embedded knowledge graphs
that we're looking at doing here
are a huge part of the delivery that
up the agent that is our solution
alone, which is kind of interesting.
Almost everybody that's doing agent-based
work agents themselves to other
agents, and you've got this compounding
effort on, which is which is powerful.
And we don't e we haven't even seen
the beginning of the potential there.
Dan Mitchell: That's exciting.
Yeah, I, you know, when I think about
LLMs and I try and compare that to agents.
You mentioned the memory required.
So having that idea of you need to
have memory of things I've told you
before as an agent, almost comparing
it to an employee trainee, right?
They come new to the job, you say,
these are the rules, you know, that
you have to work these hours, you have
to do these things, and and then you
need to come back tomorrow and do it.
You can't forget everything you just
did today that I trained you on.
And then you start to learn
and you get better at your job.
So I think that's an important point.
like, if I were to compare that
to an LLM, like a chat GPT or a
Claude, I can go interact with them.
And today I can have them do some things.
I can have it create a Word doc for me.
So how is it different from a traditional
LLM that I would interact with?
Drew Dimmick: Well, I'll take that one.
So the, the use cases that we're
looking at there, there's a couple
of constraints in the overall
ecosystem that we're involved in.
So, any.
Financial services or large it
organization is reticent to export their
data and have it preserved in the context
of any of the large language models.
In fact they actively
try and prohibit that.
So they want to take advantage of the
training and the work that the large
models are done, then it may do some fine
tuning and get a slice of that model to
use internally, but they're not feeding
that information back and they see that
as an information security risk actually.
and and a data governance risk that
needs to be really closely monitored.
So that's kind of given us impetus to
look at other ways for us to do that.
And conveniently, the ag agent
approach with the memory facilities
that we have inside of our system
allows the customers to completely
control all that memory information.
Within their four walls whether that
be in a VPC on a cloud or on a on-prem
system, that's kind of irrelevant,
but it's under their control.
And so the risk of data
scape is much, much less.
And it lends to the notion of
agentic topologies in general
because wanna have separation of
concerns for those kinds of things.
And that is a needs will be a continued
pattern inside of the enterprise.
That goes back to good practices
for data security across most
enterprise environments is,
you know, zero trust models.
Only people that have access, have
need can get access to things and
just do that from a design footprint.
And AG agentic models need to be
following that in order to be successful
inside of large enterprises, but
they're also quite powerful and
delivering all these capabilities.
Dan Mitchell: Yeah.
And you know, I just kind of thinking
about, and we're gonna get into a
little bit later in the in the, the
podcast, we're gonna get into kind
of the ramifications of agents and
liabilities and all those aspects.
But Viv, do you wanna talk a
little bit about some of the
applications that we're seeing out
there, or potential applications.
Viby Jacob: Absolutely.
I think like, just to close
on Drew's point absolutely.
Like, you know, the LLM is where we are
just responding to a prompt like a single
step sort of a process, but the agent is
far more complex interactions that follow.
And Drew alluded to pretty much all
the different aspects like memory
as well as security, as well as
making the data leaks, et cetera.
Which become even more important and
prevalent in the age of ai, agent ai.
Right.
I think on the application side,
what I would say is like we read in
the industry about Morgan Stanley's
internal advisor agent, right?
Where you're like getting.
Financial analysts with supporting agents,
supporting them with complex queries.
We read about like software tools like
PR agent that conduct code reviews.
So we are seeing a lot of these
implementations are not simple theoretical
constructs, but more of like operational
systems that are driving efficiency
gains that are measurable and also where
mistakes carry real consequences, right?
More or less the industry is pointing
that like most of the efficiencies
as well as that come from these
applications are in specialized
domains where the stakes are highest.
One big example that's being
quoted is like Toyota's multi-agent
system claiming reduction in
production planning by 71%, right?
That's a huge number.
Diagnostic assistance in clinic, clinical
decisions augmenting expert judgment.
So I'm curious as to, these are sort
of like what we read in the industry
as well as sort of like being quoted.
to hear more from Knee to end drew us to
some of the examples that they're seeing.
Then that would be one area that we
could explore in terms of what are
some of the real world as well as,
especially in the IT world, what
are some of the examples that we are
actually seeing being operationalized?
Dan Mitchell: Yeah, that, and I'd also
like to hear about some of, where you see
it going in the future, like some of the
Viby Jacob: Mm-hmm.
Dan Mitchell: dreaming,
Viby Jacob: Yep.
Dan Mitchell: What could it possibly
do in the future type thing.
But we'll get to that.
So, yeah.
You know, let's hear
some real world examples.
I mean, startup people talk to tons of
prospects, customers friendly faces,
where do you hear these things going?
Where are people thinking
about applying these agents?
How do you see them solving the problems?
And what is the benefit for them?
I.
Neetu Pathak: So from my perspective,
there are two places where
people are trying to use agents.
One where either redu automating
it reduces the time it takes
to get a certain thing done, or
humans are unable to do that work.
Even if you put a lot of, like
for example, if you have to send
personalized email today, if a person
has to do that each email might
probably take 30 to 40 minutes.
And even then it'll not be personalized.
But if you create an agent that goes
and scrape a web, kind of creates a
profile of the person that they're.
Sending to have an idea about
what kind of this profile would
respond to and then create an email
that's just code running 24 7.
But a human cannot do that.
The agents are coming handy where you
either want to reduce the time it takes
to deliver something or increase the
revenue that comes out of it, even
if there is a little bit of mistake.
Like even if you make five person
mistake out of a hundred people, five
person didn't like the personalized
email you sent, but you are getting
nine five, that's still better
than industry standard right now.
Dan Mitchell: Yeah.
And that's an interesting one
because you know in that the
stakes are not super high, right?
So if it does make a mistake and you
have a 5% error of margin, that's
okay because it just means that
maybe you didn't get that opportunity
to sell to that person, right?
Neetu Pathak: so yeah.
In that I would say, okay, so I
was talking to this another company
that is com customer support.
And basically AI is doing
customer support, but people
don't like talking to ai, right?
So you have to pretend that
you're not ai, like you have to
have a very novel conversation.
In that scenario, that 5% is not okay.
You want to reduce it even further
because if 5% people know that you're
talking to ai, they'll tell other people
that, Hey, this company is using ai.
Uh, so it really depends upon use
case to use case and what you're
trying to achieve using agent ai.
Viby Jacob: I agree with that.
You know, the most recent example is
like cursor having a moment, right?
Having a agent, customer
support, and users actually
complaining about it, right?
A whole thread suddenly spins
up and leads to even a bit of a
dent on the reputation, right?
Dan Mitchell: Yeah.
What about you, drew?
What?
What are you hearing?
Drew Dimmick: We're hearing it for, from
our initial customers with our solution.
they want to have an agent AI solution
looking at their it portfolio.
And these are things like
components and moving parts and
pieces inside of their portfolio.
It's constantly changing.
And then you have another impetus
into that whole circuit, which
is all of the standards and
procurement activities are going on.
You may have a vendor gets
deprecated, another one gets added.
You may also have security concerns around
certain versions being vulnerable or an,
or have have toxic licenses, for example.
And these are all things that people that
are building out enterprise applications
have to deal with on a day-to-day basis.
It's really arcane, slow, hard to do
work that's necessary, and it creates
huge inefficiencies inside of their
develop, development and value
delivery chains they don't do it right.
So, for example, one of our customers
had a POS system going into place.
And they weren't keeping track of
their software supply chain and all
of the different aspects of that.
And it turns out that one of the
components that they had inside embedded
in the system, was going obsolete.
And when they got to release time, they
didn't know that it was going obsolete
until they just were about to ship it.
And then they had to pull back and go
back and completely rewrite their stack
because it was a very, very essential
part of their component architecture.
And, so that probably cost them months
in development time to go remediate that.
Our approach is that hey, let's automate
all that stuff and have it done, you
know, on demand or nightly or weekly.
Take a look at where you are with
your development stuff, compare it
to your standards, compare it to your
vulnerabilities and software supply
chains and contracts, and make sure that.
You what you're building
is gonna be shippable.
That's just one of the use cases, but
this one is huge amounts of savings.
We, by our estimates, it's somewhere
around 80% savings compared to the
typical work that's done in this.
Just doing the data
gathering and the doing.
The comparison of that is, is takes weeks.
So if people don't do it well
then they end up getting that
problem when they go to ship.
So we want to help avoid those
problems and prevent those kind of
issues when they're going to market
Dan Mitchell: Right.
And, and to, uh, NI's point
they work around the clock.
Right.
You
Drew Dimmick: all the, all the time.
Right.
Dan Mitchell: you don't have
to worry about agents and shit.
Well, I mean, eventually they
may self-organize into a union.
You never know, right?
But we'll see.
We'll see how that goes.
Um.
Neetu Pathak: But when Drew was
talking about data gathering
and, and this LMS looking, I just
remember a very funny conversation.
So I've had conversations with people
who do nine to five jobs, right?
And there's this constant fear that they
cannot talk on the Slack channels or
emails that freely anymore because LMS can
consume all the data and much easier to
figure out what you said before you didn't
care because like, oh, they're collecting
so much data, be just too difficult
for them to go through all of it.
But now you cannot have personal
conversations on work forum anymore.
Uh, so yeah, it is making a lot of
difference in our day-to-day life.
Our habits are changing.
We are just not aware about it.
Dan Mitchell: Yeah unless the LLM
decides to jump in on the conversation
since they start teaching LLMs
effective use of gifs and emojis
yeah, I think they'll be part of it.
may be the most popular even.
It's hard to say.
Neetu Pathak: Yeah,
Dan Mitchell: Yeah.
Neetu Pathak: the moment you can
personalize them, there'll be
the sweetheart everyone needs.
They're all a, acting as a
therapist for a lot of people, so.
Dan Mitchell: Yeah, as long as
they don't claim to be licensed
like that that one instance there.
But yeah.
Yeah.
So talked a lot about where
we're solving problems today.
You know, futuristic view, right?
Smart cities, even higher stakes would be
AI in defense, if we could imagine that.
I don't know if we're ready
to trust it quite there.
Creative is still very, very
controversial, I would say.
Because the argument and mostly from
the artists is that, it can't come up
with anything it hasn't already seen.
And I would argue that
neither can humans but.
idea, and I think I was at
an IDC conference a year ago.
They kind of painted this picture
of personal assistance, right?
And the idea that you could have
this assistant that has access to
everything in your life and it knows
your preference on where you want
to sit on the plane and what you're
willing to spend those constraints.
But then also can map out how
long it takes for you to walk
from one spot to the other.
So it doesn't order the Uber for you
until you get a little bit closer
and then it orders that for you.
I think that with all that potential,
risk is that we become maybe not too
dependent, but maybe a little bit lazy.
Once it starts doing everything for us.
A lot of people talk
about it unlocking new.
Time and availability for us.
but I don't know.
I don't know.
Um, you know, if you've seen the
movie Wally, it's kids movie.
That's a little scary for me floating
around in a chair drinking soft drinks and
outer space while everything goes to hell.
And I'm like, is that what agents
are gonna be in the future?
What do you think?
Neetu Pathak: There are like
couple of points to unpack.
You had a lot of things right?
The, once we are successful agents,
what the future would look like.
But if we come today like what would be
the immediate future if we can create
something good enough with agents?
Um, so actually I had a thought
and I kind of made lost it.
Drew, do you wanna take it?
And maybe I'll come back.
Drew Dimmick: Sure.
Hopefully I didn't lose my thought.
I do that all the time.
so, I, see agents we're
all busy people, right?
And in, in our target customers
we're doing, but even in my own life,
I, you know, I think of the travel
assistant one, when we're booking a
family trip I've got a full-time job.
I'm doing a startup.
You know, I've got a lot of
stressors in my personal life.
And so when you get to doing those
kind of menial or travel planning, like
things, know, while it might've been
fun in the past when I had less stresses
on my life, it's not fun right now.
You just want to get it done and you
want something that truly understands,
you know, all of the things that you're
looking for and take care of that for you.
in our our resource constrained
environments, having something
like an assistant, being able to
do that for you reliably is super.
Attractive.
And I think that will be quite
beneficial for society in general
because there we don't have enough
people to get the work done.
And so, that's, you know, having an
assistant be able to do those kinds of
things and then have people doing higher
order work is much more desirable.
So, for example, if I don't have to
deal with travel planning, I have all
sorts of time that I can spend thinking
about something far more creative and
far more interesting, that, that's
much more beneficial to myself or my
family or the company I'm working for.
Neetu Pathak: So.
Drew Dimmick: I see agents largely
taking busy work out of people's lives.
I think that's the first
thing that it will do for us.
And then certainly they'll get more
intelligent and more capable, but we're
also gonna be getting more capable.
Neetu Pathak: Now Dan I
remember what I was thinking.
So when you were talking
about consumer, right?
You kind of talked about two
different things like in art.
So mostly art is for entertainment, right?
And the other one was convenient.
So you're talking about AI system
as convenient and wherever art and
creativity is coming is for entertainment.
And one of the places I was
kind of blown away, but.
Imagination of people is when the
Ghibli thing came out of OpenAI, right?
I read this one comment on Reddit and they
were like, oh, soon we will be able to
take any movie and ask it to be converted
into a certain style and I can watch the
same movie in a hundred different styles.
That was mind blowing and that is not
something that can be done by human.
So you are consuming the same plot,
but with different expression,
different kind of people.
You can even change the character
that you want that person to play.
So that's a different way of
thinking about entertainment.
The other that obviously, uh, both Drew
and you kind of data about the AI system
doing the task so that we don't have to do
manual stuff like ordering things on time,
taking calling a plumber, making sure that
everything is fine after they're gone.
All the small, small things
that we do on a daily basis.
Like if that work gets taken
away, we'll have a lot of time.
And the last thing he said that, oh,
we might be in now world like Wally.
Technically we can be, but with all
the geopolitical things that we have
today, it's very hard where a government
is paying for you to just relax.
That is never going to have happen
in pretty much any part of the world.
So that means if you want to have a job,
you have to find a way to stand out.
Everyone gets a job because there,
there is a demand and there is supply.
So if everyone is using ai, what can you
do different that produces better results
with AI that anyone else cannot do?
So I think people will get
creative because we need jobs.
So yes, we'll get lazy in certain
part of the life, but at the end
of the day, we still need to work.
And I don't know what that
kind of jobs would look like.
For instance, when YouTube
and everything came around,
influencers became a job, right?
That was not a job.
20, 30 years ago, people found
a way to make money out of it.
So we'll see some more
creative jobs coming out of it.
Dan Mitchell: That I agree with.
Definitely, definitely.
What are you thinking?
Vi.
Viby Jacob: I think there have
been plenty of innovations and
inventions in the past that have
taken time out from the system, right?
And I believe in the human tenacity,
resilience as well as like intelligence
as to how we overcame those, whenever
those sort of like time saving measures,
et cetera have happened, we have evolved,
actually we have become more resilient,
creative, intelligent, et cetera, right?
So is the human as well as agent
coexistence is what I think as more
augmentation of the human rather than
a replacement of the human . Wally was
a great movie, but I think that really
exists as like the bookend of utopia.
So, uh, that's the way I see it,
Dan Mitchell: Wally
worked very hard as a, uh,
Viby Jacob: right?
Dan Mitchell: uh,
Viby Jacob: Robot?
Dan Mitchell: know, as a robot agent.
So,
Viby Jacob: Yeah.
Dan Mitchell: Okay, changing
gears a little bit let's get
a little bit into technology.
First we've talked about agents and
what they are, but the idea of them
working together, and where they
can work and how they work together.
Yeah.
I would assume that you can have them
work together like real people, right?
Because the idea is that they're
supposed to be like people as
agents and figure things out, right?
So they should be able to work together.
Correct.
Okay.
So recently we saw a couple of
announcements and I think maybe
everybody's on the same page
that they need to work together.
Anthropic released this
concept of MCP, right?
MCP came out.
It doesn't mean everybody was all in it,
but next thing you know, it's blowing
up all over X and then there's this
massive proliferation of MCP servers.
This guy nuttle his
website, playbooks.com,
he's got 3,900 servers in a
directory on that website, right?
So what's so great about MCP and what
does it actually do for the agents?
Drew Dimmick: So it's a, it
was a key enabler for us.
We we were actually building
our own concept like MCP until
MCP came along and then all of
a sudden we're like, yeah, sure.
We're just gonna pivot over to using that.
'cause it makes a lot of sense.
and I would reference some work
done by Octo Tools which I think
is underrecognized in the MCP
success story, with the concept
of tool cards in particular.
And May, and maybe it was somebody
earlier than Octa Tools, but the
first time I saw that was with our.
with Octo, having those tool cards
available to you so that you can go
get data from systems and then process
that through LLM is actually, a key
enabler for any sort of agentic solution.
Because without that context, you
mentioned that earlier, Dan you,
and that's context to me of, real
data that's coming from real stuff,
whether that be travel data or
enterprise ID data, or you name it.
It's all real data about
what's going on in the world.
You can't really make an
agent solution successful.
So MCP unlocks that kind of
real data context for us.
A two A is interesting because now it
gives us a standard way of interfacing
with other autonomous agents.
So MCP is all about getting data
and interacting with the data.
MCP or A to a is really more about
how do I interact with other agents?
And this is also work that we had in
our roadmap that we wanted to get into
because we see our system itself is
gonna be an agent to others we'll be.
like the subservient chicken, fine.
A lot of vendors don't want to be that.
But new modern vendors will absolutely
wanna be consumed by others as agents.
And so we're off to doing our work
independently and autonomously.
You set us up to do all that work, and
then we can feed and interact with other
agents who may benefit from our work.
That's a fantastic scalable approach
to designing modern systems.
So, I think a two A is, you know,
a welcome entry here it's taking a
lot of bespoke work that a lot of
people were doing independent of one
another and making it standardized.
Viby Jacob: so your point is it's not so
much as to MCP or not to MCPM, CCP and A
two A are complimentary, MCP allows you
to connect to the content repositories,
dev and environ, et cetera, and a two A
Drew Dimmick: Yeah.
Viby Jacob: the multiple different agents
together to form more of a multi-agent.
So the two coexist in
Drew Dimmick: Yeah.
Viby Jacob: okay?
Drew Dimmick: So I'm making an
agent that's really good at doing X.
You're making an agent that's really good
at doing y and another person's making
another agent that might wanna need X
and Y and add them together, or, cross
multiply and divide, whatever, right?
And, and that's we can't predict
what that's gonna look like, and
that's actually what we're unlocking.
So this is a fantastic architectural
approach to unlocking enormous value
by letting these autonomous systems do
the things that they're really good at.
And it's going to require,
vendors to get on board.
Unfortunately, a lot of the legacy
vendors don't wanna get into the A to
a thing because of the threat to their,
platform models and, bringing people
onto their platforms and trying to
entrap or en encase their data and in
boats, that's there's gonna be resistance
in the legacy to their detriment by
the way, they will they'll end up
getting surpassed by modern entrance.
Viby Jacob: So with, so within
Dan Mitchell: Go.
Go ahead.
Viby Jacob: you know, would you say
like the security are the constraints
that you mentioned earlier, right?
Are those managed or.
Contained by the enterprise
or by, is there any support
Drew Dimmick: Oh
Viby Jacob: or from A two A or is it
like the enterprise that's devising,
that's putting together these agents
are solely responsible for, all
the security data leak, et cetera?
How do you have to,
Drew Dimmick: is always a shared
concern, especially in the enterprise.
You, everybody is responsible for
security, to the call center agent all
the way up to the c CEO and the C-suite.
That's the way good
security practices happen.
When you're deploying assistance,
it's no different, right?
So whether you had a human assistant
or an AI assistant they need to
be handling data appropriately.
Our approach, MCP has, a new solution.
It's not unexpected to see novel
attacks and novel approaches to
using it to do nefarious things
or potentially nefarious things.
If there's potentially some design
improvements that could be done there.
I've been particularly concerned that the
observability and auditability of what
an agent or an MCP, connection is doing
is a critical concern for the enterprise.
They need to know what these things
are up to for audit and compliance
purposes, as well as security purposes.
And then the identity and control and
access controls, needs to be addressed.
We have a very simple approach.
Concept there, because our MCP approach
is that a user having the agent do work
for them, doesn't get access to data.
They wouldn't have access if
they went directly to the system.
So we're not trying to create
an overlay additional privilege
access management layer.
We're using the existing privilege
access management layer that's in the
underlying data source to do that for us.
And that's a good lightweight concept
that makes MCP lot simpler to implement.
But other MCP approaches are trying
to implement an entire privilege
access, authorization layer inside of
the MCP server itself, which is gonna
be duplicative of what all of these
data sources already have and gonna
create holes and gaps and coverage
and unintended consequences there.
So think we've got some
architectural work to do.
We've gotta go back to the gym.
make that more mature.
But Mc p's moving so fast.
It started with you know, standard out
in input output, got went to server
side events and that was in, into
streaming HT TP and, and in a month.
It's, it's the, and, and OAuth is now
put into to context and on and on and
on, like the inva innovation here.
People are listening the community.
I see a community of people doing
this development is just amazing.
And, we're just drafting on that
tailwind because don't have the,
we're five people in this startup.
I can't go build something as big
as this, but this community is built
something which is just awesome.
Neetu Pathak: So I, I wanted to
kind of draw on the history of MCP.
So actually MCP was create
open sourced in November.
Obviously atropic might be
using internally before that.
The reason it blew up is.
First, a lot of articles started coming
out of A 16 C and other talking about
model context protocol that, so whenever
a VC firm starts writing something, it
reaches the startups directly, right?
Because they're kind
of keeping track of it.
The second thing that happened that
actually blew up is the announcement that
OpenAI and Gemini might start using it.
So there were a lot of tool
calling softwares out there.
MCP was not the only one, but there was
no standardized approach that everyone
would be using this one technology.
Suddenly the fact that MCP had approval
from most of the biggest player, that's
why it drew up, because now people were
like, okay, even if it doesn't per work
perfectly, the fact that everyone else is
using it and the community as a whole is
contributing to it, I can depend on it.
I can create my software around it.
It's not gonna break because this is
going to keep getting better and better.
Uh, the other thing I wanna
say, just a distinction.
So MCP is think about a code.
That directly talks to your
databases that can receive, prompt
and return you back something.
So one of the reason people like
MCP is there's a deterministic
approach to your MCP server that
otherwise is lost in agent to agent.
So if you are asking another agent,
Hey, can you tell me what my sales
quota looked like last quarter?
You cannot have 2.1
or 2.5
if your thing was 2.6
million, right?
You need to have accurate data.
So where wherever you want
accuracy, MCP becomes a thing.
So in, in the best world, you'll have
an agent that always refers to an MCP to
get their data needs and the other agent
ask this agent so that that data can be
combined in different ways based on the
context or the prompt they're receiving.
So that's where a two A comes in
and MCP is just to make sure that
you don't mess up data that you're
get getting from different places.
Drew Dimmick: I completely
agree with that.
Me too.
That's, and also both of them just
have great three letter acronyms.
Dan Mitchell: yes.
And so, yeah, just for the, uh, for
the, the folks listening the alphabet
soup we just threw at you, MCP stands
for model context protocol and a
two A, as you would imagine is agent
two, agent the two in the middle.
Right.
But you bring up an interesting point that
there's there's definitely a halo effect
when you see broad adoption of a standard.
think somebody told me this week
that there's something like 15 other.
Options to MCP, but because we're already
down the path and people are able to adopt
it very quickly, and it with a pretty low
barrier to technical entry, especially
if you're using coding assistance it
seems very, very easy from what I've
observed to be able to implement it.
So I guess I could see how that would
drive it, even though these announcements,
they seem a little bit superficial to me.
But fine.
It is what it is.
Right.
Okay.
Shifting gears again let's talk
a little bit about the ethical
implications and challenges agents.
Right.
So one thing I'll mention again.
So there is this.
Paper that was published it's
authored by Margaret Mitchell and
Ava, gosh, both of hugging face.
And basically what it says is we should
not produce fully autonomous agents.
So that's kind of a counterpoint to
what we've been hearing where people
talk about, okay, we'll start with
human in the loop and then we'll
have human on the loop, which is
the idea of supervising a team of
agents, but going fully autonomous.
We have clashing views here.
I dunno what you've heard, Viby, but,
I mean, it scares me a little growing
up in the era of movies where robots
take over the world, but that could
just be a generational thing for me.
But at the same time, I could see how
having fully autonomous agents who are
performing tasks that you don't feel like
there's a lot of risk could be beneficial.
What do you think?
Viby Jacob: I think the
paper it's a great paper.
I think what the paper suggests
is that like we need to.
Distinguish between levels of
autonomy, levels of agents, right?
Similar to any other autonomous systems
like we have SAE levels in autonomous
vehicles and robotics, et cetera, right?
The more control that you give to a
non-human entity, there is also the risk
of there is more risks involved, right?
So the paper suggests.
Look, there are different types of agents.
We need to sort of like set a taxonomy
for the agent autonomy so that like we can
understand the risks better and also put
in control mechanisms to man to manage it.
And also like how do we verify some
of the safety related aspects, right?
So in order to drive those aspects, the
paper is making a claim towards the fact
that there is no clear benefit only coming
out from fully autonomous AI agents.
We need to, there's also foreseeable
harm that exists with those, so
we need to manage them better.
Right.
That is the call to action
coming out from that paper.
Um, need to, and drew, uh,
your thoughts on those.
Neetu Pathak: So I, um.
See agents are not that different at,
if you give agents a complete autonomy
and in a perfect world, they're
logical, they're not that different
from human, that is given a task.
You every human needs check-in balances.
You cannot ask a human to go, you
know, give them $10,000 and ask
them to do something and assume that
they're going to only buy work stuff.
There's a reason that you want race
a you, somebody has to go through it.
It's the same thing with agents.
So having an autonomous agent do
something without check-in balances,
obviously it's not gonna work.
If you have the right check-in
balances, then it shouldn't
be that much of a problem.
The only difference though, is we
as humans, when we grow up, we have
a lot of environment conditioning.
Like the fact that we understand, well,
this is ethical and this is not ethical.
Or for, like, I, I gave
a very simple example.
So suppose if you have an agent
that is supposed to avoid churn.
If it learns that it doesn't show
people who churn in the report,
then the churn rate is low.
I mean, it achieved its goal, right?
But that's not an
ethical way to do things.
So for an agent to understand those small
nuances that we human do, that's why
they are more dangerous than a human is.
And you'll always need some kind of human
oversight no matter how smart they get.
Just because the way we perceive the
world and the way we want to exist in
this world is going to be always different
than the way agents exist in the world.
Drew, what do you think?
Drew Dimmick: I totally agree.
You know, my mental model on this
is probably a little simpler.
I don't think auto autonomous, truly
autonomous agents really exist.
There's always some sort
of human in the loop.
It's just how many layers of the
onion out are you from that, and the
feedback the controls that you would
have on those agents needs to be clear.
But there is always going to be the checks
and balances that you mentioned me to.
May be some may, maybe in some cases
that's an accident investigation, right?
Like that's the sad, unhappy path, right?
Because something went wrong
in the way some agent was
behaving in a, in a vehicle.
You know, but the, having the
advantages of having these autonomous
agents probably vastly outweigh
the disadvantages or the concerns
provided that the controls are there.
Dan Mitchell: Well, ni too,
you made a interesting point.
Like, so when you grow up, right,
you've got this notion of ethics, and
maybe some people don't, but for the
majority of humans, I believe that the
majority of humans are good, right?
So if I were equate, if I were to equate
that to raising a young person, right?
So the agent is my young person.
The responsibility, the accountability.
So if you're raising a child
and the child does something
dumb, the parent is accountable.
Right.
So if we get to a point where these
agents are working in a fairly
autonomous environment and they screw
something up, who is accountable?
Neetu Pathak: So I have heard a lot
of different founders come up with
different ideas, and I think the one
I liked the most was the fact that
they should be an insurance company.
So because I mean, having a kid and
a parent is a very different thing.
But in a world where everything is
based on ROI, my agent is working with
another company's agent, and if it makes
a mistake, there is an insurance company
that pays for it like PayPal, right?
So it, it paid the merchant before
receiving the money, and if something
went wrong, they kind of managed both
the, it's like a broker kind of thing
That makes more sense than the ethical
consideration of who's gonna take a blame.
Because I don't think anyone is gonna
take a blame when agents go, Hey, is it
the company that's providing the model?
Today's element, it can be something else.
Or is it the data it was trained on?
Is it the prompt that someone wrote?
It's a very hard question, and I
think the easiest way would be to
have an insurance broker in between.
Dan Mitchell: It's a, it's a novel idea.
Drew Dimmick: It's a great,
yeah, it's a, it's a great idea.
Um, I would say that having worked in
the insurance business for a little bit,
there will always be lawyers underneath
it trying to assign the liabilities to
the various piece parts and, and that's.
of our legal system and, and, uh, and
the accountabilities that it builds.
So I would expect that these are
considerations that need to be
put into place and we should be
conscious of, the potential risks.
And that, that's, so our opinion as
we're building out this thing is, it
is we're providing soft output reports
and, insights into enterprise data.
We could take actions, right?
But we are taking a wait and c approach
there because a, the risk tolerance of
our customers may not accept us going and
making changes to live production systems
based on information that we discern.
As they get more comfortable with it.
Will they do that?
I am pretty sure they will.
How long is that gonna take?
We love to say that AI is
moving really, really fast.
But I think that, if you look at
autonomous driving and the complexity
there and how long it's taken us,
and the lack of completion of that
you know, our, all of the starry-eyed
prognosticators that we're saying it
was gonna happen in a year, and we're
all gonna be driven to work by, by,
by our car, and we can have a cup of
coffee and relax, hasn't happened yet.
not reliably and not, to
the level that people are.
So I think that adoption is gonna
have a long curve ahead of us.
These systems are of equal
complexity that I deal with.
I think other human systems are
gonna be of equal complexity.
But there's tremendous opportunity for us
to all learn and really build that better.
Viby Jacob: If I, I, if I sum up like
the agents have transformational value
we need to have governance frameworks
like need to mention insurance or like
other liability measures, et cetera.
Have the proper organizational operational
culture and frameworks in place.
And then also tailor the business problems
that are e amenable to the risk posture
for a agent-based approach, like you
mentioned, drew, based on the risk profile
or the appetite that they have currently.
Right.
And then evolve over time,
depending on how technology,
as well as frameworks evolve.
the gist that I'm hearing.
In terms of like, let's not
get bogged down by the, you
know, all the risks, et cetera.
Let's look at what's possible and
let's kind of like move with optimism.
Dan Mitchell: No matter what happens or
what we might think we want to happen with
agents and liability, everything is gonna
be figured out at that first court case.
Where, the agent ends up in court
and it's trying to defend itself
through an LLM conversation.
it may hallucinate in there
even though it's under oath.
It's hard to say.
But I think that whatever, whatever
happens there that's gonna set
precedent and then we'll just
all have to follow suit anyway.
Neetu Pathak: The one thing that you,
Dan, so the fact that when humans say
something that's incorrect, either they're
ignorant or they're lying, but LLM is
always hallucinating so it can strategy.
Dan Mitchell: That's right, that's right.
In fact, the LLM will have to
swear on perplexity that, that
it, that it is speaking the
truth and nothing but the truth.
So verified and perplexity.
Neetu Pathak: Hall.
Viby Jacob: Right?
Dan Mitchell: Yeah, it's true.
It does.
It does.
Drew Dimmick: and, and, and you'll
get a different answer tomorrow.
So I.
Dan Mitchell: So, so where
do we wanna go from here?
Viby.
What, what's next?
Like, we got a little bit of
time left with these folks.
Um, you know, the, the future,
you know, what do you think?
Viby Jacob: I think like, one,
one question I have is like,
you know, there are so many
applications that you mentioned
from agentic AI perspective, right?
If we look at the AI world we
kind of like, here are three
or four top use cases, right?
Content generation, code generation,
customer support, et cetera, right?
Within agent ai, do you see anything
that's sort of like popping up?
Like, you know, top three five, your top
three five, where you think, the most
common agent AI applications would be?
Neetu Pathak: So one trend that
I'm seeing is use of voice ai.
So obviously you can talk a lot
faster than you can type, and you can
read a lot faster than you can hear.
And that is taking, obviously people
haven't figured out, but there are
a lot of things that are coming
up to increase human productivity.
Like instead of typing, you're
speaking and then you're just
seeing the responses back.
But according to me, that will make the
change into something called invisible ui.
So right now we have a
lot of websites, right?
We, if I want to find an article,
I have to go and search it and I
have to click a couple of buttons.
Sometimes I have to Google,
Hey, how do I go and do this?
That wouldn't exist.
Your entire website could be recreated
or shown based on your conversations.
If I want to cancel.
My subscription, you know,
I can, Hey, how do I cancel?
It brings up the page that I want.
There'll still be some visual elements,
but what I'm seeing, I don't see
us clicking through everything.
And it also might be possible
that the webpage design
itself can be very different.
What I see versus what you
see might be very different.
And that's in a different era
of how the businesses are
done and everything is sold.
The lines between marketing, sales,
creative writing, engineering
product, everything is going to blur.
Um, yeah, I'm very clear about
that and I do see that changing the
entire life cycle of the product.
Its itself.
Viby Jacob: Very interesting.
Drew your thoughts.
Drew Dimmick: Actually really similar.
I think it is helping us accelerate
you know, where human, our human
frailties or whatever you want to call
it, are are getting in the way of us
really progressing as fast as we can.
And so when you talk about you know,
reading and speed of reading and I think
those are gonna help accelerate the.
The human condition, it's gonna, it's
gonna come out in a number of different
forms and things that I can't wait
to see what this community innovates
on because it's all to, to be seen.
We're, we are just at the
very tip of the iceberg this.
So, I'm excited to see it, but I, I
don't dare say where it's gonna go,
Dan Mitchell: It's probably
Drew Dimmick: I wanna, I, but I
wanna be on the iceberg, right?
Dan Mitchell: Yeah, definitely.
We would rather be on the iceberg
. So yeah, I think, last one,
uh, humans and agents, right?
Talked a little bit about will
humans retain control of agents?
I think they'll try you know,
and do as best they can.
We'll have this concept of humans
managing agents as companies get leaner
and they get into you know, especially
for like the task worker model.
You can have a bunch of agents
doing that work, but they
still need some supervision.
David Linthicum, well-known cloud
guy also into a lot of AI stuff.
Now.
He said the other day, is
there an ROI in agents?
And it got me thinking a little bit
about, well, it depends on how expensive
the it is, or how cheap it is for a
human to perform a task, So we went
through this whole era of, outsourcing
and offshoring and trying to drive
down the cost of technology, right?
And as we know, are
still pretty expensive.
So if you take a use case like a coding
assistant, or you can generate lot of
code and it's relatively good, does it
save you the money of the coding assistant
doing the job of that developer, and now
you don't have to pay that developer.
Or maybe you can do it with
two developers instead of 10.
Okay.
Fair.
Right, because again, developers are
expensive, call center agents as a kind
of counterpoint are not as expensive.
So are you gonna see the same ROI
or are you gonna see sufficient ROI
for moving to those types of agents?
If you were to replace call
center representatives with agents
Drew Dimmick: yeah, uh, I can, uh, so,
uh, there's one company I know about from
our customer interviews, what they're
telling me a story about their use of ai.
For con call centers actually in
particular, and there was absolutely
return on investment there.
it actually flips the
equation of their claim.
It was a claims processing workflow and
use case that involved a lot of paper
and receipts and things like that.
So I think like corporate
expense reports kind of stuff.
they flipped the ratio from 95%
manual handling of those things
to 95% automated by using.
An AI design system that is
essentially an ag agentic AI system.
What's the human implication there?
Right?
You have contact centers and
people doing paperwork, task
work that are high turnover.
The least.
I mean, is they turned over people
every four months on average.
You know, you can't, people
can't keep people in these jobs,
so they're able to do the work.
Then they might have needed, a hundred
people and now they need 10, right?
they flip the ratio they're
able to do with 10 people.
Those 10 people are making a little more
money 'cause they're actually doing higher
value work and ha and handling the true
escalations, better and more aggressively.
So custom, meanwhile the customer
experience is going up into the right.
So ROI.
Is a really interesting thing.
Right.
And it actually comes
back to most enterprises.
That's where I'm working in, don't
really do full, full bore TCO analysis
that would drive their ROI setups and
they tend to be cherry-picking in a
lot of cases and it becomes political.
And so I would say yes for sure.
There are use cases that
have ROI absolutely.
Money back guarantee.
Neetu Pathak: So,
Drew Dimmick: Is it universal?
No.
Neetu Pathak: but then I'll take it
more from a psychology perspective.
Even now, if you see there are certain
use cases where people don't wanna
talk to other human in contact center.
If I have to see my bill, I would rather
log in than call and phone, right?
And even if I'm calling a phone, I'll
probably select one to hear my bill.
I wouldn't wanna talk to a person.
The reason I wanna talk to a person
is because whatever constrained
choices they have for me, first I
have to listen through all the press,
one for this, press two for this.
And it might not even, I might go through
all that thing and find out that they
don't have answer to what I'm looking for.
And that's when I wanna go to human,
because I feel it's gonna be faster.
Or they might have certain
information that the compromised
version is not gonna have.
So if you can flip the script, like if
that when I'm calling someone can give
me all the answers that I need and it's
accurate, we are becoming very as humans,
we are actually becoming more introvert.
We don't wanna talk to other person.
People don't take calls,
people like messaging.
So we will prefer not to talk to human
unless we feel like the humans have
certain information for instance, okay.
If there is a, so, so something got
just launched, there is a good chance
that the people in customer support
doesn't know about that product as
much yet, or the bugs as much yet.
So the agent can do that much
better because it's just, you
know, they get the data and they
have all the recent knowledge.
Human people need to be trained for it.
But if I feel like, oh, something really
bad is happening in the com company
and they really need to understand from
a human, get some kind of inclination
what might be happening, I will probably
call a customer agent because they might
know if there is something down or they
might know from other conversation what
might be happening so they can co-create
assumptions that software systems cannot.
So yeah, I mean, there'll
be a huge ROI just to switch
away for whatever questions.
We don't actually need to talk to a human.
Everything else can be, uh, automated.
Dan Mitchell: you're talking customer
satisfaction, which drives ROI
because it's customer retention,
it's the right, it's efficiency.
People are happier overall,
will recommend your company.
Drew Dimmick: Yep.
Dan Mitchell: I could see that.
Drew Dimmick: Well, and there's really
strong evidence, Dan going back into the
nineties when I was helping with contact
center and tech support stuff, customers
back then didn't want to talk to people.
They actually would rather have
read, on our uh, dating myself.
But they would rather go into a bold
board system and see a tech note, right?
To see what, how to solve.
They wanna self solve.
They don't want to interact
with other people because
they're trying to move at speed.
And the talking to a human means
you're sitting in an on hold queue.
You're wasting time.
You have to get them up to
speed on what's going on.
Half hour later you're still not
getting an answer that you want.
And that's, if you can do a lot
of stuff in self-service, it is
better in general for the customer.
And that overall, ROI, which to me is
related to TCO becomes much higher.
Totally agree.
Me to like, and the
psychology hasn't changed.
I don't think it's actually changed
as much as you're asserting.
That would be the only place I would
push back a little bit as I think this
has been human behavior for a long time.
Dan Mitchell: I, it could be a
little bit generational, it could be
side effect of COVID and Covid kit.
It could be a few different
things, but no I mean, just to
share a quick anecdote with you.
So, the other day I needed to call
the pharmacy about a prescription
refill that they had the prescription
on file and every other time.
Time that I've called the automated
system hasn't been able to help me, right?
Because it has no idea
that this is on file.
It doesn't understand that concept.
They had introduced at this pharmacy
the notion of, oh, well if you can't
be helped, you can leave a message.
So you don't have to talk to a person.
You can leave a message and it
will forward that message to the
pharmacist and then you can choose
to get a call back or not if they're
able to solve your problem entirely.
what happened this last time when
I called was something different.
So in the past I had sent the voice
message and then I got a text saying,
oh, your prescription's being filled.
No problem.
This time it sent me back a
summary of the message that I left.
So that meant that at
some point, some system.
what that was and pass that
along to me and presumably pass
it along to the pharmacist.
So we're seeing little iterative
improvements in these systems
where there's this interpretation
of the communication you're
trying to send forward.
And so I, that was kind of a nice
feature that, yeah, to that point, it
did make me want to keep going with that
pharmacy because it got a little bit
easier, . Well, we've taken up a lot of
your time, we really appreciate here.
I think that we'll probably wrap up.
You know, today we talked about ag
Gentech, ai, we talked about its
applications, ethical concerns.
What is it gonna look like in the future?
Any kind of parting
thoughts before we wrap up?
Drew Dimmick: I'm looking, I need to, um,
so, uh, I think underlying psychology here
is really interesting to, to unpack that.
I think we look at the underlying
human motivations here and
why agents are attractive.
And then Dan, your example to me
is really important uh, improperly
or implemented agent, like what
you interacted with your pharmacy.
And I think I know what the pharmacy is.
Um, it's horrible.
It drives people away and it
makes people sour on automation.
So That approach agentic AI to do tasks
like that because they're with so much
knowledge and ability and context,
unlike any system, you know, an IVR
system that's ever existed on the
planet customer experiences will be much
higher and I think the tolerance for
interaction with agents will go up higher
because the experiences will be better.
Dan Mitchell: Okay.
Me too.
Anything.
Neetu Pathak: I mean, I know this
is a very controversial topic
where people feel like agents
are gonna take away their jobs.
It always happens when the
new technology comes along.
But that the thing is.
These concerns will be there till we
start seeing new jobs that are getting
created when these agents are taking
away jobs and use the situation.
There's always this in-between time where
you don't know the new jobs are coming
and you're losing the existing ones.
So yeah, I mean we, I don't know what
kind of jobs will come in future.
It'll be very interesting to see
probably working with agents when it
hallucinates or oversighting agents.
I don't know.
Maybe it'll allow people to write
novels that were really bad at
writing or create children books
that they couldn't do before.
So what is gonna change?
And I don't know what looks like,
but humans will always have jobs.
I don't think we, I see a future
where we will not have jobs and
agents are doing everything.
Dan Mitchell: That's great insight.
Viby, why don't you wrap us up?
Viby Jacob: I think it
was a great discussion.
We started with agentic AI definition.
Some of the characteristics, how
it differ, differs from standard
automation, which is like a,
rules-based heuristic sort of approach
some of the leading applications.
We heard differing thoughts on,
the path forward for agent ai.
consensus around the fact that we
need to look at the potential of
AI agents rather than the risks
associated with fully autonomous agents
and you know, go from there, right?
And really put in place the governance
frameworks, the control mechanisms, et
cetera, that's necessary to harness the
potential out of any invention, any tool.
In this case, AI agents, right?
some of the examples that were mentioned
were very realistic as well as like, you
know, something that everybody can relate
to, including a pharmacy example, right?
So I think it, it for almost comes
down to this, if we like factor
in the labor economics, the ROI.
Provide a path for
implementation of ai agents.
It can take you know, that there
is massive transformative potential
associated with it, and we
definitely should get on with it.
Right?
Not so much as a job replacement who
thought about prompt engineering as
a job like five years back, right?
None of us con, contemplated that.
So there is definitely like shift in
economics, in labor economics that might
come about, like job descriptions and
things like that, but certainly an avenue
that we should you know, go forth with.
I see.
Drew Dimmick: And now we're,
and now we're automate.
Now we're automating prompt engineering.
So there you go.
Viby Jacob: go.
Drew Dimmick: That was,
that was a quick job.
Viby Jacob: Yep.
Dan Mitchell: Alright,
Viby Jacob: Very fast
moving world, but with a
Drew Dimmick: yep.
Dan Mitchell: excellent.
Well, I wanna thank Nito and
Drew for coming and joining us.
I'm Dan Mitchell, my co-host, Viby Jacob,
if you enjoyed this conversation today,
please make sure to subscribe so you can
hear future episodes and we'll drop a
teaser on what our next episode will be.
But for now, this is mainly ai.
Thanks.
Drew Dimmick: Thank you, Dan.
Thank you.
VI.
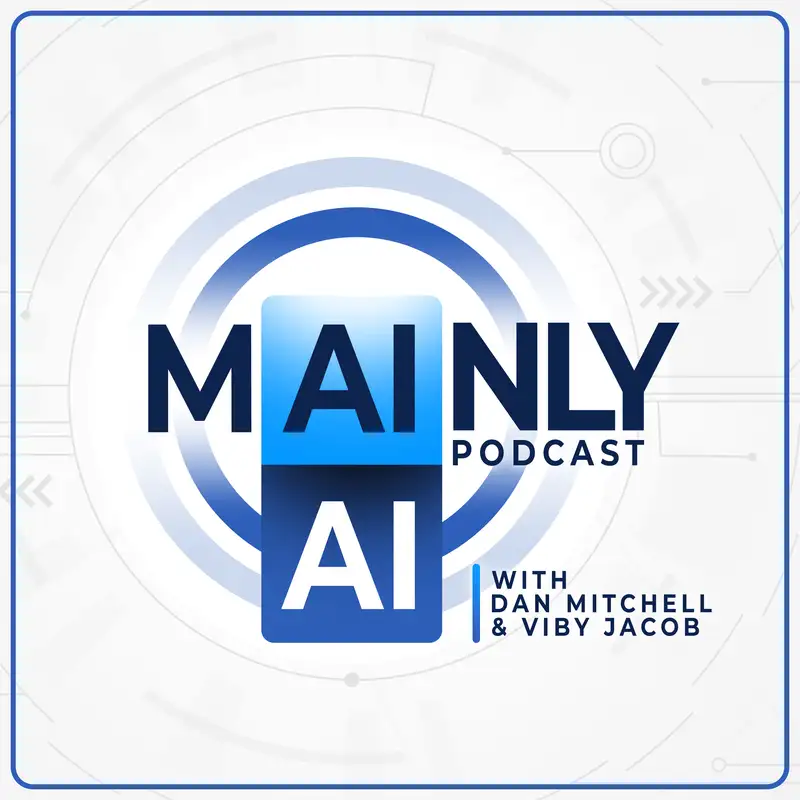